How to maximize the ROI of RWD
Optum experts give a snapshot of commonly encountered pitfalls when using RWD and recommendations to help avoid them in this PMSA webinar.
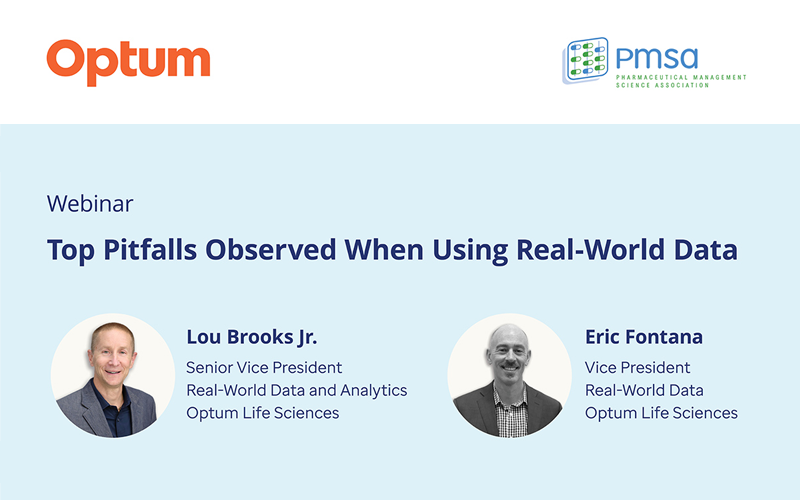
- High, selected
- Low
- captions off, selected
- English for the hard of hearing Captions
This is a modal window.
Top Pitfalls When Using Real-World Data | Optum
- [Chris] With the webinar. Thank you for attending today. Your lines are muted, but if you have any questions during today's presentation, please type them into the Q and A panel in the Zoom platform, and we'll answer them at the end of the presentation. Today's topic is "Top Pitfalls Observed When Using Real World Data," and will be presented by Optum Life Sciences. I'll now turn things over to Eric Fontana and Lou Brooks.
- [Lou] Thank you, Chris.
- [Eric] Thank you very much, Chris. Good afternoon, everyone, on the East Coast, and good morning to those west of this time zone. Thank you for taking time outta your busy day to join us for our discussion, "Avoiding Common Pitfalls When Working With Real World Data." Now, we've got a pretty good size crowd on the line today. I know a few folks are probably still making their way into the webinar, as is custom at the beginning of these things, dealing with perhaps a little bit of new tech for everyone. So while you all get situated and we allow a few later attendees, a few introductory notes. First of all, thank you to PMSA for hosting us today. We'll start off with some introductions. My name is Eric Fontana. I am the Vice President of Client Solutions with Optum Life Sciences. I'll be your co-host today. Of course, when I say co-host, that means I'll be ably joined by another colleague. So I'm pleased to introduce my colleague, Louis Brooks, the Senior Vice President of Real World Data and Analytics here at Optum Life Sciences. Lou, welcome and thank you for bringing your expertise to today's discussion.
- [Lou] Eric, as always, it's a pleasure. You know how much I enjoy hanging out with you, so hopefully, we can be a useful tandem today in providing some folks some of our perspectives on how you can work with real world data a little more efficiently and maximize your return on those investments. So looking forward to spending the next hour with you and everyone on the line.
- [Eric] Thank you, mate; I appreciate that. Well, a little bit of background. For those of you wondering who Optum Life Science is and what we do, we are a division of Optum Insight. Our mission is devoted to helping life science organizations move quickly from insight to action through a combination of data, consulting, and tools. Lou and I both serve in the data licensing portion of the business, specifically dealing in healthcare claims, Electronic Health Record, and integrated data. By integrated data, I mean the unification of claims with eligibility controls and Electronic Health Record data to provide perspective into both the healthcare transaction and the underlying clinical detail associated with a patient encounter; a best-in-class real world data substrate for researchers, data scientists, and epidemiologists to conduct analyses, supporting a wide range of applications within life sciences. And for more information, if you would like to, you can go to optum.com, look under the Life Sciences section in the drop-down, and you'll be able to read a little bit more about us. So let's move on to the subject matter of today's discussion. And maybe it's appropriate to ask in the beginning, why are we here today, and what are we gonna talk about? So this is our road map for today's session for the topic of "Avoiding Common Pitfalls Associated With Real World Data." Lou, this discussion has been a long time in the making. The team that you and I oversee work very closely with numerous life science organizations of vastly different sizes and levels of experience, working and really interacting with real world data. And I think through those interactions, we've observed some recurring challenges. So borrowing from the philosophy that one should learn from the lessons of others, we've boiled down a set of the most common challenges we observe. And I wanna just lay down a few pieces of detail that should provide some helpful context for the discussion. The focus of today's session is particularly pertinent, I think, for frontline data users and managers. And we'll be presenting an executive perspective on this topic in November at STAT in Boston. If you happen to be heading there, please come and say hello. But for today, three parts to our discussion. We're gonna start out with the why, and we'll provide a little bit of background on how the executives we speak with tell us that they're thinking about real world data, because there is some important context for everyone on the line today to know. Second part, we'll move into the meat of the discussion, talking about some of the common pitfalls when working with real world data. And we're gonna do our best to be solution-oriented in this section. So rather than just dole out the challenges we observe or admire the problems from 10,000 feet, we will really try to tease apart some suggestions for how to short-circuit some of these problems earlier upstream. And then in the final part of our discussion today, we'll wrap it up with some closing thoughts and recommendations. That'll include a short set of diagnostic questions that you can run yourself to get a sense for whether your organization is on track. Now, what this webinar will be and won't be; I think this is some important detail here. It will be about the common challenges we've observed as we supported our clients working with real world data. It won't be about real world evidence generation, per se, not about statistical techniques or approaches to generating evidence. And while those are all excellent discussions to have, what we're talking about today is probably far further upstream from that; some would say maybe more fundamental, but really, really critical. I also just wanna clarify here that the type of real world data that we're talking about today specifically pertains to claims, Electronic Health Record, and integrated details. So we're not necessarily speaking directly to sources such as registry data or other external activities not routinely captured in those sources we'll be talking about, although some of the lessons we'll talk about today no doubt could apply, if you wanted to think about them in a similar vein. One last note, we will address any questions at the end of the webinar, so please pop the questions into the chat panel if you have some, and we'll pull them up at the end and go through. So if that sounds good to everyone, and the caveats are understood, I say we charge ahead. Now, I wanna kick things off here with a little bit of detail on how the executives we've been speaking with over the last eight to 10 months think about real world data. And I know everyone on the line is probably pretty hungry to hear about the pitfalls, but this is an important piece of insight to begin on. And the pitfalls that we'll talk about are candidly a barrier to achieving some of these goals. So, Lou, I'm gonna turn to you here. Both you and I spend a significant amount of time with senior leadership at life science organizations, both executives directly in the real world data space and those maybe more removed from the day-to-day use, but definitely involved in the procurement or financing. And it turns out, especially this year, two overarching themes have dominated our discussion. There is one theme on the Return On Investment of real world data. And then, consistent with the documentation many of the folks probably saw on the line back in September of last year, the recommendations from the FDA on real world data, the requirement for sponsors to adequately explain the data used in the research. So, Lou, I'd love it if you could describe a little bit more about what you're hearing with regard to these two challenges.
- [Lou] Yeah, certainly, Eric. I think the first, in ROI, I think, as we continue to look at the data landscape, more sources becoming available, data's becoming fractured as more organizations are looking to enter into this particular space. And, frankly, executives are struggling to try to figure out what is the value of the purchases, licenses that they have today relative to these new and growing sets of sources. And what we've learned is that there aren't a lot of organizations that have well-established methodologies for evaluating Return On Investments of these particular assets that they are acquiring. And they want to get there. They're being asked questions around some of these investments, 'cause data licensing is not an inexpensive activity, in many cases, spending tens of millions of dollars on data, and really not having a great idea of what that is bringing about in terms of value. And so they're looking for ways to tighten that up. They wanna move away from simple utilization metrics to really gaining a true understanding of, yeah, we've done X amount of work with this particular data asset, we were able to glean that this generated Y amount of incremental benefit to the business, whether it's cost savings or incremental revenue. And, yes, this is an asset that we need to continue to make part of our foundation from an analytics perspective, because it is generating value. And I think we're gonna see that continue to grow. We're gonna see the sophistication of organizations continue to evolve and move in that direction as they continue to get pressed on the budgets that they have for real world data. And I think with the FDA, that's been a huge amount of energy that's been expended on discussing that with clients. It's unclear what it means truly for all of us, both from the sponsor side as well as from the data provider side. It's guidance at this point; it's not anything formal. But many of the data assets that we've grown accustomed to leveraging over time don't necessarily meet all of the criteria that have been outlined in those guidelines. And that's gonna require all of us to take a hard look at how we acquire and construct these research data assets, how transparent data organizations are with sponsors, what types of auditing and data providence modifications are gonna be required. So it's an exciting time, because I think it speaks to the fact that the FDA is looking beyond just established clinical trial data to draw conclusions and make decisions on. But it does open up a fair amount of work that will need to be done to ensure that we're all prepared to provide the same level of data providence that the FDA is looking for in a real world setting, as we've all gotten accustomed to in producing on the clinical trial side of things.
- [Eric] Thanks, Lou. Well, look, let's unpack the ROI concern in a little bit more detail here on the next slide. And I think it's fair to say that ROI perhaps is a vexing challenge for many life science organizations. All of the executives, as you'll recall, that we speak with, they want to do it better, and yet there is, at the same time, a palpable sense of, depending on who you talk to, frustration, while simply not really having a good handle on how to move forward. And I think if I was to characterize what we've been hearing and observing, there is how life science organizations are doing it today, and then there is an aspirational state that many of them would like to get to. So on this slide here, we've summarized, very broadly speaking, what today looks like at many organizations. And I think we've also taken a crack at what an aspirational state might look like based on some of our own research. Lou, ROI seems conceptually simple on the surface; I think you've alluded to that in the prior slide there, and yet many still struggle with it. What's your take on where most organizations are today versus where their executives would ideally want to get to in the future?
- [Lou] Yeah, Eric, it's a great question. And I think the biggest challenge today is that many organizations still have a fairly fractured and siloed method for acquiring and disseminating, and even using the data. And in the conversations with various organizations, what we've seen is that the organizations that are closer to that aspirational state have centralized much, if not all, of that function within one organization. And, as a result of that, they're better able to work through some of the challenges on methodology, on collecting all of the uses that are occurring with the data, with specifically controlling how data is being acquired. So they're not either double-paying for data or buying data sets that are extremely similar with each other, but different groups are ultimately acquiring them. And I think that's ultimately one of the key factors that organizations really have to think about if they truly want to get to ROI. I mean, I remember in one of the sessions that we had where we got into the conversation around one organization just having different views and measures of ROI, depending on what part of their organization they talk to. And without having some type of common framework, you never really get to a point where everyone agrees that this is the true value that we are arriving at from utilizing the data. And I think that's ultimately where folks want to go. I think, today, counting the uses, how many publications, dossiers, et cetera, we've used the data for is a solid proxy that everyone can feel good about. But it's gonna take a little more of a concerted effort to get to that true ROI calculation, if that's where organizations truly wanna be.
- [Eric] Thank you, Lou; that's great insight. Now, let's really briefly touch on the second top of mind issue, those FDA-related concerns. And to recap, I mean, really ensuring that sponsors can understand and explain data to the FDA. And, Lou, I'll cut to the chase on this one. We've both read the recommendations in detail that came out in September of 2021, basically detailing the responsibility of sponsors, sponsors being the life science organizations conducting research, have to understand and explain the real world data being employed in their analyses in detail. And I'd love to get your take on why this is such a concern for life science executives and what the implications are for them.
- [Lou] Yeah, I mean, this is one of those pieces that, as I said a moment ago, I've spent a lot of time talking to folks about. I mean, we've ultimately had to modify some of our contractual agreements with clients to make them more comfortable. I think the major concern here is being left holding the bag. The sponsors are accustomed to running a clinical trial and having full control over the data from which that trial generates. And when you start working with real world data as a sponsor, you've got access to the data, but you don't have access to the machine that generates that data, the processes. You don't have the data providence; you don't have all of the quality controls. You built all of that within your own organization. You have an extreme level of comfort with it. And that's ultimately what the FDA is looking at, to a certain extent, on the real world data side, and that's where the concern lies. Because sponsors are a step, or in some cases, maybe even two steps, depending on where the data's coming from, from how that data was originally generated, and that causes a lot of trepidation for them. They don't want to be left, let's call it, left at the altar without a partner to be able to educate the FDA on the various questions around data providence. And it raises the bar for sponsors in making sure that the data that they're using is of sound foundation and can be appropriately shared should questions come up. And I think that that's something that all data organizations are going to have to really take a hard look at as we look to support our life science clients to ensure that we can give them that same level of comfort and clarity that they have today with their own clinical data. And that's really what this boils down to. A lot of what's in those guidelines is steeped in that historical use of clinical data, and we're gonna need to figure out what that right balance is.
- [Eric] Yeah, that's a fantastic point. And I think for folks on the line today, you will see, on this slide, a small sampling of some of the questions life science organizations, admittedly at a high level, would need to ask and be well-versed in, and certainly could go much deeper than that. So with the Return On Investment and FDA concerns for executives in the rearview mirror now, I wanna talk about six factors or pitfalls that we've identified. And perhaps not coincidentally, all of them exacerbate the challenges of achieving ROI, either directly or indirectly, and certainly are signs and symptoms of organizations who have either knowledge gaps or are not necessarily approaching the use of the data in the most efficient manner. So I'll go through each of them quickly here, and then we can unpack them in detail. But as you can see by the header, where we see life science organizations not using data that is fit for purpose, not understanding that medicine usually isn't practiced like a clinical trial, not appreciating that all information on patient interactions isn't documented in claims; not recognizing the variation in treatment and outcomes is not uncommon, or perhaps, is common. Not starting data investigations through the lens of a specific research question, and not adequately considering patient privacy risks when linking data with a de-identified asset. So as I mentioned a little bit earlier, not diving into sort of the evidence generation, but some of these fundamental challenges that we see well upstream of that. So let's dive into each of these in detail, and we'll start out with the first pitfall. Maybe this seems rudimentary, fundamental, to many on the line, but there's a reason we bring that up, "not using data that is fit for purpose." And when I think about that phrase, "not using data that's fit for purpose," it's kind of like the old "wrong tool for the wrong job." Maybe like trying to, say, bang nails in with a wrench. You might almost be able to use the data, but the ultimate outcome won't necessarily be as successful as if you'd employed the hammer. And often a yellow flag phrase I think we hear when we work with clients is that the data isn't really matching with our expectations. And the thing that can make the data set not fit for purpose are many reasons. It could be the wrong population representation or an incomplete population representation. It could be inadequately capturing the site of service that the particular therapeutic area need included, or using claims detail when clinical detail, such as that found in the EHR or maybe even integrated data is a better choice. And I really wanna be clear about this one. Often this one gets conflated with a data quality issue. But, to us, this is really a "you didn't spend enough time upfront asking the right questions" issue. So you'll see here on the top right, we've got some very generic examples that hopefully illustrate what we are describing in more detail. But, Lou, I'd like to get your take on this. What types of recommendations are you typically making to analysts to avoid this fit-for-purpose problem?
- [Lou] Yeah, this, Eric, is something that I think we need to spend more time on as a data organization. And this is something that we are certainly shifting from a mindset standpoint. It's really pressing hard on what are the key elements that are necessary to meet the needs of the business questions that are being asked? So if there are specific clinical measures, biomarkers, lab results, financial metrics, whatever the case may be, make sure that you've compiled that list of "these are the essential elements" and push on the data that you are looking to use to address that question. And make sure that you have, in the course of working through that list, the must-haves, the nice-to-haves, and you're working with whomever's helping you assess a particular data asset to ensure that you've got all of that information. I think the other thing to do is to make sure that you plan appropriately. A lot of times, you run into these challenges as well, because midstream, we decide that something else might be interesting. But in midstream, it's not the time to make that particular decision. And I know that can be difficult. I mean, I'm a researcher myself, from my old days early in my career, and I've lived that particular problem as well. But I think from our perspective, what we would recommend is ensuring that you really plan out what the essential data elements are, and you really pressure-test those against the data that you want to utilize. And that may or may not mean a data set's appropriate. It may mean you may or may not have that data set available to you today, and it may mean that you need to alter what the analysis is going to be able to do if you can't get your hands on that information. But it all starts upfront with really planning and assessing what you truly need.
- [Eric] Thanks, Lou. And I can't reemphasize that point that you made enough. I think the over-investment in understanding exactly what the data is capable of doing for a particular project is a really critical one. So onto our second pitfall, medicine isn't usually practiced like a clinical trial. I can hear some of the clinicians on the line probably giggling in the background. This one I can speak to with a little bit of personal experience from days past in clinical practice as well. Sometimes researchers may reach out asking why, say, specific pain scales or disability scores or functionality assessments are not broadly contained in the clinical data. And often this is because clinicians are not performing those tests or assessments in a routine manner. So unlike a clinical trial, which has very well-defined parameters and data collection for analyses and routine clinical practice, is typically focused on catering towards the patient, and often, as a consumer. And the nature of the clinical interaction is very different. Lou, roughly how many times in your career have you heard a researcher lament that the specific elements they're looking for, be it pain scores or functionality scores, specific outcomes of interest, patient-reported details, just to name a few, are not resident in the data, and the researchers just can't understand why.
- [Lou] More times than I care to admit to, to be honest. And it's a frustrating piece for researchers from that standpoint. I mean, there are objectives that they're trying to achieve from that particular standpoint, but it's something that can be addressed. I think part of this, and this is a common theme that Eric mentioned, he obviously has a clinical background. We added him to the team last year for this particular reason. It's easy when you're a statistician or econometrician data scientist. You're working with the data, you're experts in models and things of that nature, and you learn a certain level of expertise on the clinical side of things just as a result of all the research and the work that you do, but you're not a clinician, and can't speak highly enough of making sure that you bring clinicians into the mix. Not only when you're laying out your objectives from a research perspective with real world data, but as consultants on the project when you're doing it. And I know we all have KOLs, right? We all bring them in. As much as we love them, I would say they're not always the right answer either. Because they're doing things, in many cases, maybe a bit differently than the rest of the market. The rest of the world may be operating in a slightly different way. And so getting some folks that have true clinical experience, they've been in patient care, and having them available helps to facilitate this particular pitfall. And that, I can't speak enough about, from that standpoint, because they're the ones that will tell you WHY those pain scores may not show up, based on the complexity of calculating them. Or more importantly, they'll tell you what they do do in clinical practice, and you can look at the data and mine it to find those pieces, and then build those scores in a slightly different manner, rather than grabbing them directly from an EMR or another data source from that particular standpoint. And that's, I think, something that we all need to be cognizant of, that making sure that we do have that clinical perspective helps us avoid this particular pitfall as we work with real world data.
- [Eric] Yeah, I really love that that point that you hit pretty hard on there, Lou. I think bringing it back to the perspective that the nature of day-to-day clinical practice is, in many cases, very different from the clinical trial. That doesn't make the data unusable. It puts the onus on the researcher to come up with a way to develop maybe proxy or using some of those elements where specific outcomes are desired. But I wanted to also touch on one other element here. The other insight is one I know you're actually quite passionate about, which is the provider collaboratives. Could you maybe talk a little bit about why you think so highly about the possibility for provider collaboratives, and what they could do to bridge some gaps here?
- [Lou] Well, I think we all speak from the lens at which we look at healthcare, and those lenses don't always line up. And I think there's a way for us to do that by getting, I mean, it's not just providers, it's obviously life science companies, even payers, ultimately working together in, and on some of these unique challenges to facilitate some of this information flow, and ultimately influence changes. I mean, I remember, is a project that our team is currently engaged in with a life science company provider organization and our sister insurance company on lipidemia guidelines. And even today, with all of the work that's been done and all of the activity that occurs in hyperlipidemia, still a large percentage of patients that are not treated according to guidelines. And, can we influence that? Can we work through that? And as we're piloting this with this provider organization, the answer that we found is yes. Sometimes it's just helping to connect the dots from a data perspective and engaging providers in the dialogue. We have to figure out how to do that in a very efficient manner, 'cause they do have patients to care for. But if we can solve for some of those pieces by getting together to work on these problems as a group rather than independently, we can not only educate each other but also improve the delivery of healthcare and the outcomes associated with it.
- [Eric] Thank you, Lou; that's awesome. Let's move ahead now to pitfall number three. This is one that, candidly, I perhaps wish I had a dollar for every time someone asked me this one. "Why don't these claims contain all the detail we need?" And I think this one is probably a pitfall maybe fair to say more commonly seen for researchers in the earlier phase of their career, but occasionally, we see more experienced researchers getting tripped up here as well. And I think it's important to recognize that claims are, by their very design, a financial record of an encounter. And I think it's fair to say that while claims are often drawn on heavily as part of research, both on the provider side and in the life sciences, they were never really designed with real world research in mind. Now, from my experience, I think it's really important for researchers especially to understand where the claims come from, what they contain and what they represent. Primarily, these are designed to support how providers are reimbursed. So while they're very good at including information to support transactions, often miss some elements that would help give a fuller picture of the patient. And I'll give you some examples. Imaging often isn't captured in inpatient claims, for example, because it doesn't inflect DRG-based reimbursement. In ambulatory claims is another example, primarily based on HCPCS CPT codes that drive the fee-for-service-based reimbursement, you could miss some of the diagnostic profile of the patient because, again, that doesn't always drive reimbursement, obviously, depending on the payment model, if we're talking capitation or fee-for-service. And while we'd all like to see other elements like social determinants of health via z-scores, I think it's probably fair to say until they are intrinsically linked to reimbursement, I probably remain skeptical that we'll see them documented in a more comprehensive manner across-the-board. Now, I do wanna be clear, claims can and are, or can be and are, a fantastic substrate for a lot of analytical exercises, but it is important to understand they reflect financial transactions, and to consider that accordingly. Lou, apologies it took me a minute to get to you here. I will get down off my soapbox here. But what recommendations do you typically give when it comes to the criticism of claims not having the full detail that researchers are looking for?
- [Lou] I know we focus this pitfall on claims, but I think it goes just a step beyond that as well. I think it applies to just any data source, EHR, PROs, whatever you're collecting or obtaining from a real world data standpoint. And I know I'm gonna sound like a broken record, but, you constantly remind me of that, but it's okay, it's about planning. It's about, again, laying out what your key objectives are and lining up the data source, and understanding the flow of data. Just like claims is missing certain pieces of information, Electronic Medical Record and Electronic Health Record data has the same challenges. Those challenges tend to be focused on things like fulfillment of prescriptions and cost information. And it's easy to miss from that perspective if you don't lay out a clear plan for the study upfront, and really line up the data source to meet the needs of that particular study. And so, again, I'll strongly encourage everyone to really work on how they plan and how they interrogate what data they're going to use before they get started. And, look, there are ways to link data together. Obviously, organizations like HealthVerity, Datavant, Viva, amongst others, have various technologies to enable the linkage of data. And part of the ability to link that data, and we'll talk a little bit about this in a moment, has its own pitfalls. But nonetheless, it has a lot of promise for enabling you to reduce the missing pieces of information and filling in gaps that you might need for a particular research study. And so I would also encourage folks to consider that as an option. It's gonna add some time to it, because you've gotta make sure the result and data set is de-identified, but by planning and opening yourself up to the potential for joining disparate assets together, you may be able to get that complete set of information that you're looking for in order to address all of your questions on that particular research problem.
- [Eric] Brilliant; thank you so much. I appreciate that insight there. Let's kick ahead, then, to pitfall number four. A common refrain from researchers we sometimes get is that they're observing something in the data but that doesn't necessarily square with what they'd expect. And I think those expectations could seemingly fall short to the researchers in numerous different ways. More commonly, as you'll see in some of the examples that we've got here, we get questions about, for example, why a surgical rate for a specific intervention doesn't match expected rates, or why a certain type of patient receives maybe a, one I heard recently is an example, an incorrect diagnosis based on the data presented. Of course, the questions we get asked are not only about things that seemingly happened, sometimes these things are about the care pathway steps that didn't happen. And all of this really boils into sort of a broader category that we might put here as not understanding that physicians and patients don't always behave as you would expect. On the clinical side, or on the provider side, I should say, this sometimes falls into a category, you might have heard this term bandied about, called "care variation." And it's important to note that variation is more common than you'd think, and it's also driven by a multitude of factors that could range from choices that, say, the physician consciously makes, all the way through to many of those behavioral economic type factors associated with healthcare, where a patient may, for example, choose to seek care or not seek care for a variety of reasons. And I think we see often this consideration sometimes trips researchers up a little bit. Lou, again, I wanna probe your mind on this one a little bit because it's a big challenge for some researchers as they start to explore real world data. What's your take on, firstly, physician and patient behavior and how that reflects in real world data? And then maybe you can give some recommendations specifically to the audience on how to overcome the variation that they should expect to observe in the data.
- [Lou] This is perhaps the most challenging, I think, of the pitfalls to kind of work your way through, because there is this expectation, right? We have guidelines; we have clinical guidelines for diseases. We have progression for diseases and recommendations on how to treat that progression. And, yeah, that exists, but it's just like anything else, right? It's a norm, it's a goal, it's an expectation, but it's not the way things play out, because we are not, and we do not all operate as rational individuals. On the clinician side, the clinician is obviously looking at an individual patient and all the factors and information that they know, and they're making the best decision, as you know, Eric, from your days in clinical practice, to treat that particular patient in that particular time. And we're not there, right? We don't know what is going on in that particular treatment room. And so it's difficult to second-guess that. And patients, look, we all don't do things that we're supposed to do when our doctors tell us to. We may not go fill that script; we may not go get that test; we may not feel any different, so we stop something. I mean, all of that stuff happens. You have to think about it from your own individual lens when you think about what's in the data. And all of that is taking place. The variability is actually something good, at least in terms of learning about what's going on in the market, and it gives you something to work towards in terms of a goal. But I think you have to be very focused and curious on understanding why that variation may be occurring; again, connecting with clinicians and others, to talk to them about their own experiences and correlate that. I remember a project we did a number of years ago, I won't mention the disease area or the product itself, but we were doing a persistency study, and the expectation was that once patients started taking this product, they just wouldn't stop, because it's for a life-saving product from that particular standpoint, and struggled with our client at the time working through that particular project. And it wasn't until I grabbed a physician, a well-respected physician in this particular disease area, brought them into the conversation, and they rattled off five or six different reasons why this individual physician would stop a patient on this drug, side effects, contraindications, other pieces. And we were able then to go back into the data and actually demonstrate that that's what was causing the drop in persistency that we saw in the analysis. And it opened everyone's eyes that, yeah, we may think certain things should operate in one manner, but there are a lot of things going on when you're practicing medicine, and we have to be open to that. Now, that's not to say that data errors don't exist, and you need to make sure that you are pressure-testing the data providers to ensure that they've got good quality control, good data providence, and the data that you're getting doesn't have errors. Because, look, all of these things are processes, and processes can break down from time to time. And so it is good to have that skepticism, but you do have to realize that, in most cases, in my experience, it's not been a data error that's ultimately gotten as the final result. It's typically the variability that exists in how patients and physicians practice and manage medicine.
- [Eric] Yeah, I think that's strong insight, Lou. And I think the other thing that I take away from this is, as we continue to see data sources expand and grow, and we continue to see the inclusion of broader segments of the population, some of those factors, behavioral economic in particular, go beyond the purview of just the clinical decision-making, and will need to really be understood by the researchers in order to have a good handle on why or why not certain care pathways have been followed. So there will be certainly a continued evolution of our understanding in that realm, and you definitely need to bring all experts to the table on a particular project. Pitfall number five now, moving ahead. This one, kind of a humorous characterization of number five, but this is going on, and often, contextless, a walking tour of the data to determine just how usable a given data asset is for research. Lou, you'll recall in many of our discussions with senior executives earlier this year, this is an observation they sometimes make about their own research teams, and it may affect researchers regardless of tenure. I think we've all been there before. As the analyst side of us, we get a new data set that almost has that fresh-cast smell. We crack it open and we start running counts. But at a certain point, that instinct has to kick in and cause the researcher to ask, "Wait a minute, what question am I actually trying to answer here?" And if there is no question, and the researchers find themselves just counting things, that should almost be a self-awareness moment assigned to pause and take a different approach. And the reason it's important to have that pause is because, in most instances, I think it's nearly impossible to understand what value certain data may have without a guiding question to orient the overall exploration. And so, in many situations, Lou, I know we've both seen cases where the assumption is then made that a data set is not usable, when actually it is very valuable once the broader context of the question is taken into consideration. So again, I'll throw this question to you. How big a problem do you think this is, and what recommendations have you made in your 20-plus years working with researchers in life science to help them overcome this almost instinctual pull to go on a walking tour, to guide the value proposition of the data?
- [Lou] Yeah, I think the easiest way to summarize your recommendations on this is to line up, and it hinges on some of the other pitfalls, line up the use, making sure you've got specific questions, and then leveraging the right data to answer those questions. That's the easiest way to facilitate this particular piece. 'Cause it's easy to get lost in the weeds. And you said it best with the wrench and the nail analogy earlier in the webinar. Not every data set is going to meet every need. And if you're just kinda wandering through it and randomly throwing questions at it, it's gonna be hit or miss as to whether or not you're gonna see any real value from working with that data. It's when you plan it out, you've got a specific objective in mind that you can truly evaluate whether or not the data may meet that need or not, and can allow you to quickly and efficiently move on to a better data asset for that particular question if doesn't fit the bill.
- [Eric] Excellent; all right. Lou, we're gonna take a brief detour to our next pitfall, and talk about patient privacy issues. This is, as you can probably see the one slide that kinda breaks the mold here, from the flow. But as everyone on the line can probably appreciate, our organization traffics in de-identified data assets, and this ensures patient privacy is maintained at all times. It's a top priority for our organization, and candidly, we believe it should be a top priority for all organizations working with real world data. Now, on the top left of this slide is a snapshot of HIPAA-related penalties issued by the Office of Civil Rights, or OCR, a division of Health and Human Services, both from an events and total fines standpoint. And while there are a few blips up and down, the general trend line on these fines continues to rise over time. Of course, 2022 is still in progress; we'll see where that ends up at the end of the year. And while the fines themselves in aggregate may not seem large, nevertheless, an important insight here is that almost all of the fines were due to failures to safeguard patient data, meaning none of them cover willful misuse. Now, you can click some of the links to the organizations in the blue rectangle at the bottom to read more about those cases, but that's a really important distinction. When it comes to non-health provider entities that would include, based on the sort of governmental business categorization, life sciences would fall into that, as distinct from the provider side, the FTC is clearly sending some public warnings about intentional misuse. FTC fines, by the way, or the European regulators, in the case of the Amazon fine detailed here, these tend to be much larger than those issued on the provider side today. But I think there's probably even bigger issue for many, overarching all of these financial penalties, which is a reputational hit. Nobody wants to see their organization on the front page of, say, "The Wall Street Journal" for an individual privacy breach. That's not where most executives wanna wake up to first thing in the morning on any given day of the year. The bottom line is here, I think, scrutiny on issues of individual privacy are expected to grow, both in the public consciousness and also in the government agencies that enforce them. And that brings us to pitfall number six here, which is failing to consider patient data when linking data with a de-identified asset. Lou, I'll turn this one over to you early here. As we see more and more organizations looking to drive insight by linking external sources of data to some of the real world assets we have, for example, including some cases where they're linking two de-identified assets together, what are some of the biggest things that life science companies should be keeping in mind?
- [Lou] I think the biggest and most important is that joining two de-identified data sets does not result in a de-identified data set. I think if you guys don't leave here with anything more than that, remember that, because that is important. The risk of de-identification is, as all of you know, is due to the variables that exist in that data asset. And so when you link, you should always be re-verifying de-identification. And one of the big issues that we've seen, and as much as I enjoy working with my colleagues at Datavant and HealthVerity, and elsewhere, it does open up a potential Pandora's box in our ability to control the uses of the data as we look to link. And as we continue to realize that we can do some of these things, our desire to answer questions in more detail continues to grow, we need to be very cautious about that. Because the downside risk of a violation goes frankly beyond fines. In my mind, there's the reputational risk, and then there's also the risk that organizations, such as ours or others, may decide that licensing this data is just not worth it. Putting it out there in a de-identified format isn't worth it in the grand scheme of things. So we all have a certain level of responsibility to continue to make sure that we're asking that question whenever we take a de-identified data set and we wanna do something else with it.
- [Eric] Excellent; thank you very much, Lou. This is one of those areas that I think we continue to see, as organizations have a greater appetite for insight, potential orange flags, shall we say, on the horizon. And we certainly take it very seriously, so we will be safeguarding that as best we can. Bonus pitfall time, folks. This one, as you'll notice, probably wasn't listed on the front. I was holding this one in case we had enough time, but I think we do, and this is a good one for us to go over as well. Lou, this one is using claims-based analytic techniques when working with EHR. And I know we said, at the beginning of the webinar today, limited discussion of analytical techniques. This one kinda skirts the line a little bit closely on that discussion. This is one of those record-scratch-stop moments for members of our team when they hear this. I think if there's anything that you've taken away from the prior pitfall we reviewed earlier, it should be that the claims of EHR detail can contain some very different data elements, which means you would be wise to not automatically treat them the same way from an analytical standpoint. And I'll be really candid. In distant years past, we've probably seen members of our own internal team of researchers feel like they could probably replicate a claims-based approach, perhaps like in an analysis like developing a line of therapy, and simply point the query to an EHR data source before, perhaps, Lou, I think it's fair to say, being gently redirected that we probably shouldn't do it that way. I know you've seen some great examples of situations where this has gone wrong in the past. Can you give us a high level of just how problematic assuming claims of EHR data is, or assuming it's close enough to just use the same approach?
- [Lou] Look, as embarrassing as it is, I can even admit, a decade ago, when we first gained access to EHR data, I was that same researcher. It's an easy trap to fall into, right? You've been working with claims data, you've got methods and procedures, and codes sitting, and so you go, "Look, I'm gonna go do this study," grab a new data asset, you just run it. And then all sorts of things go wrong. The job may not work properly. You get results that are inconsistent or just flat-out weird, and you're left struggling and trying to figure out why from that standpoint. And I think the easiest thing to just make sure you do is, as you look to bring in or leverage a new data asset, you ensure you take the time to understand what's in it, what's not in it, how it was constructed, what's the underlying framework for it. As Eric mentioned, as we all know, claims is payment; EMR is care delivery. And then make the appropriate modifications to your processes to accommodate the subtleties within those particular assets. I mean, it seems kinda straightforward when we explain it that way, but it's a lot harder in practice, because you've invested a lot of time and energy, you've got a significant repertoire of techniques, and you feel like you could just go run one. And that's not where you should be when you're looking at leveraging a new data asset.
- [Eric] Thanks, Lou. Okay, that gets us through the pitfalls. Let's move ahead, because I really wanna wrap up with a little bit of discussion. We've got about five minutes left before we can move to questions. And I wanna bear in mind many of the pitfalls that we just discussed. And doing that, this slide arguably might be the most important detail of the whole discussion. So we've just spent, say, 45 to 50 minutes talking about pitfalls, all of which have potentially negative implications for the two goals that we discussed at the beginning of the session. That is the ROI and the FDA considerations that executives are thinking about. So here we've provided some practical questions you can ask yourself and your organization more broadly regarding your capabilities today that should help unearth any yellow flags in terms of how you interact with real world data, and whether that might be driving suboptimal performance on those broader organizational goals. And for what it's worth, in our experience, these are questions that almost all life science organizations would benefit from reflecting on at some level. So just a broad orientation. Here on the left, we've lifted out some questions to ask your organization, and on the right, questions to ask of any potential data partners. Lou, shall we review these together, and we can sort of just bat about really briefly whether there's any additional insight to offer here. I think for those on the left, your organization thinking about defining questions ahead of time to ensure that licensing is appropriate and non-duplicative, thinking about how our staff, you know, continuous cycle of learning. Clearly, that is an underlying theme for all of the pitfalls that we discussed today that would really support ameliorating some of those challenges. Are we developing methods to investigate data specific to the needs of a given project? Are we ensuring shared learning, so that any institutional knowledge we do develop is retained, and then also accessible to others within the org? And then have we implemented policies to determine how our organization will use data-linking and mitigate risks associated with re-identification? Lou, any broad thoughts there before we look at the questions on the right?
- [Lou] No, I think they're all fairly clear. I think folks can make them how they need to be structured for their particular organization.
- [Eric] Perfect. And on the right-hand side, I think, importantly, if there's something we haven't emphasized enough here, when thinking about potential data partners, thinking about what they can bring to the table to support your advancement towards those organizational goals we outlined at the front. So, for example, are you teaching licensees to use the data well in advance of a critical business need, that upfront education? Providing easy-to-read resources that expedite learning; the FAQs, the data explainers. Where required, on-demand support to address some of those research-related data questions that we've outlined today. Of course, supporting that would be documentation with detail on QA and QC processes, how the data is actually pulled together and formed. And then having mechanisms to evaluate compliance and facilitate data-linking, so you can get through that re-identification challenge that we talked a little bit about earlier. One last thing that I wanted to wrap up on here. And as you can probably appreciate, a lot of the pitfalls we described today come from knowledge gaps. And what I mean by that, to be clear, are not knowledge gaps associated with data science or statistical techniques, but very addressable gaps associated with understanding the composition of the substrate itself. Everything from where the data comes from, what it represents, and how to think about it, effectively required learning upstream to interact with the data in an analytical fashion. And that requires reinforcement over time. So if you're looking for a data partner, it's imperative you ask them about their ability to help you get over those knowledge gaps as quickly as possible. Three characteristics we think are important to look out for. Do you have a diverse, dedicated support team to build that knowledge, both for you and of you? Does that provider have robust education and training support? That should be a given. And last, self-service resources for the routine but important questions to help speed up the process of support. That's a very high-level overview as we come to the end of our time today. Lou, any final comments that you'd like to offer on this component?
- [Lou] No, I think, let's see if we've got some questions. We're almost out of time.
- [Eric] Yes.
- [Lou] And let's get those handled.
- [Eric] So Mahia Duntalay. Mahia, you said to us, "Talk business; that's all I want from you." I don't know if that was a comment reflecting something regarding we suggested upfront, or if that was what you were asking us to do, but I hope we talked enough business today to hit that goal. We had one from an anonymous attendee, how to solve missing data problems. Lou, would you like to address any thoughts about missing, or quote/unquote, "missing data"?
- [Lou] So missing data, obviously there are various imputation techniques you can leverage depending on the analysis. You have to be very careful about whether or not you decide to impute data. I think the way that we've looked to support organizations with data that's, quote/unquote, "missing" or something that they need, is helping them find a source of that data, and then linking it back to the broader data asset in order to meet the individual objectives for that particular analysis. The challenges with data imputation, depending on the analysis that you're doing, are somewhat troublesome, and so I would utilize that as a last resort. And if all else fails, you may have to shift your analysis if the data's just not available.
- [Eric] Absolutely. Question from Andrew Right on pitfall number four, "What should researchers do to prepare to support unexpected results by being ready to address the possibility of bad data or bad coding?" Now, pitfall number four, you may recall, is not understanding physicians and patients don't behave as you expect. I really think when it comes down to looking at the results that you're getting vis-a-vis the coding, I think on the coding side, it should really be about getting a second, third, fourth set of eyes over the coding, if possible, to make sure that results that you're producing aren't off because there's been an incorrect data pool along those lines. But the possibility of bad data is an interesting process, one that we can run in a number of different directions. There's bad as in maybe it's incorrect coming from the source, which is a very different problem than I think the data not necessarily having the outcomes in the way that you would like. And that's one thing that I think is really critical to make a distinction about when you're looking at the detail, is the detail not what you would wanna see, but accurately reflective of what happened? Or have you, in some cases, manipulated it into a point where it no longer represents what it wants? And so what researchers can ultimately do is, I think this might sound very fundamental, but it's baking in a pause to ask, what are these results that we've got? Are they real? Could we check them? How could we check them? And who should check them and be involved in that? And so that's almost like a QA process in terms of research. So I don't know if you would approach that any differently or augment that.
- [Lou] Well, I think the only other thing, the phrase "bad data or bad coding," it's, to a certain extent, we have to kind of unpack that to establish exactly what we mean. But the big challenge to note is the way that I look at some of that is analyzing your data, looking at distributions, trying to identify the various outliers and scrub that data from your particular analysis from that perspective. If you truly find that the data is, in fact, bad, i.e., the organization that provided the data screwed up somewhere, then it's paramount and fundamental that they go back, make that modification, so that you can rerun the analysis with the appropriate information. But there's always going to be, quote/unquote, "bad data" in any source, because humans are entering it. Whether it's the wrong CPT code or ICD-10 code, or it's a mistype on a score or measure in an EHR, that stuff happens. And as all researchers, we do need to do a certain level of cleansing before we do our work, and we have to be cognizant that that's going to exist in real world data. I think we have one more question. I know we're over time.
- [Eric] One question, and we're over time; yep, absolutely. And hopefully, I think there's a theme in the answer that you just gave to the next question here. We got a question here from Paul regarding ICD-10 codes and CPT codes used by different provider organizations. "How consistent is their utilization of such codes "in their claims records? "And should we avoid pooling claims data for an analysis?" There's really two questions in that, to me. One ties to an answer you gave a second ago, Lou, which is basically, I think that when you start to look at human errors associated with coding, personal experience, having worked on the provider-based revenue cycle world for a significant period of time, suggests that the more closely that coding ties to dollars and reimbursement, I think you can be assured that it's more comprehensively documented. The further you get away from codes that directly lead towards reimbursement in a particular area, then you can start to get into questions about incompleteness or completeness. So that's probably one way that I would address or think about that question. The second part, Lou, I might turn to you for some of your experience here. "How should we avoid pooling claims data for an analysis?" What's your take on pooling claims?
- [Lou] So from my perspective, pooling claims eliminates the potential bias that might exist. So the more claims that you can pool, the better. I think the fundamental question behind the consistency, I think is just a function of analyzing and understanding the patient populations, right? Because if we think about something, let's say we look at something like macular degeneration, right, that's not going to be something that's gonna show up in younger patients. So if you're pooling data from organizations, and for whatever reason, you've got organizations that tend to care for younger individuals, and you're pooling that with data that's caring for the over-65 population, then, obviously, your overall results are going to be somewhat skewed in that regard, because the populations are different. So don't pool pediatric data with retiree data, as an example. But I think, in general, if you're looking at pooling large health systems, provider organizations, data integrated delivery networks, things of that nature, that the subtleties in what might be going on from a coding perspective all blended together get averaged out in the end. So I think if you approach the problem by evaluating the patient populations from which the data comes from initially, that's probably the best mechanism to evaluating whether or not you can pool two different data sets together, and make sure that you get a consistent analytic output as a result.
- [Eric] Fantastic. Lou, thank you so much for your partnership on the discussion today. And, everyone, that brings us to the conclusion of our call. I hope you found this material valuable. If you do wanna reach out to us or have questions about the material today, our email addresses are fontanae@optum.com or louis.brooks@optum.com. Thanks, everyone. Be well, and we'll see you next time.
Top pitfalls observed when using real-world data
The life sciences industry is increasingly drawing upon real-world data (RWD) to inform a host of business functions across the product life cycle. These include clinical trial support, market insight, comparative effectiveness, understanding care standards and more.
Despite the richness of insight available through EHR and claims-based data assets, we sometimes encounter concerns from end users about data quality and its ability to support the analytic goals of an organization. These concerns are often the result of misconceptions around what RWD reflects versus the desired analytic purpose.
During this PMSA webinar, our experts provide a useful snapshot of some commonly encountered pitfalls and recommendations to help avoid them.
Let us help you connect the dots
From data to insight to action, we catalyze innovation and commercial impact. Have questions about EHR data? Contact us today.
Related content
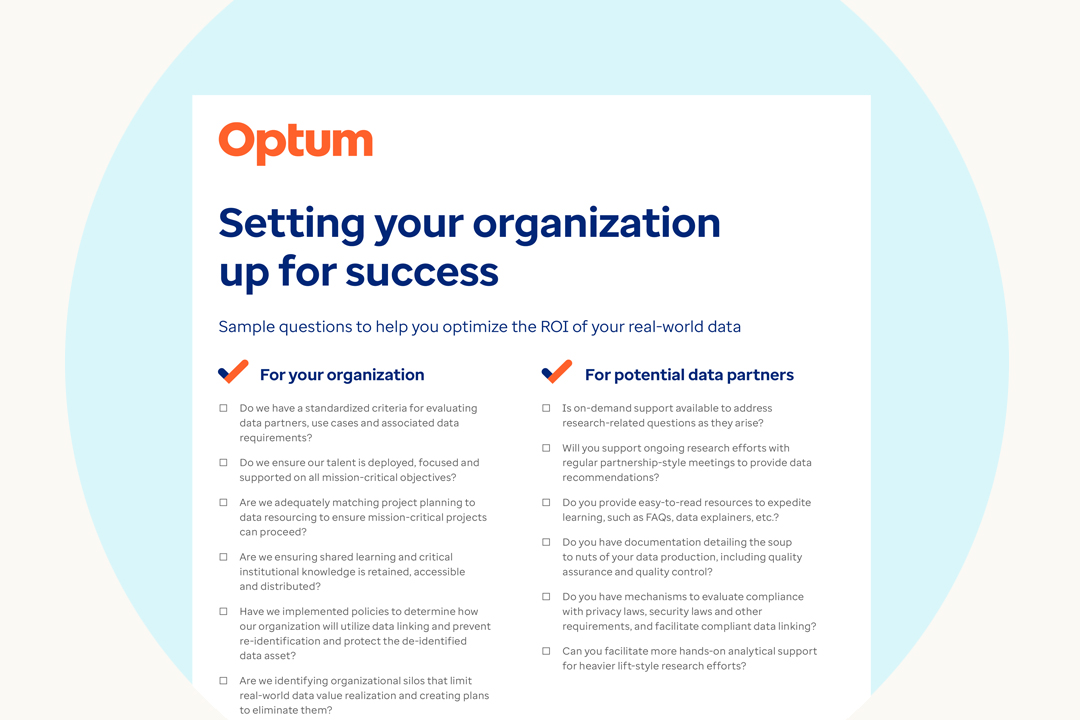
Setting your organization up for success
Check out sample questions to help you optimize the ROI of your real-world data.
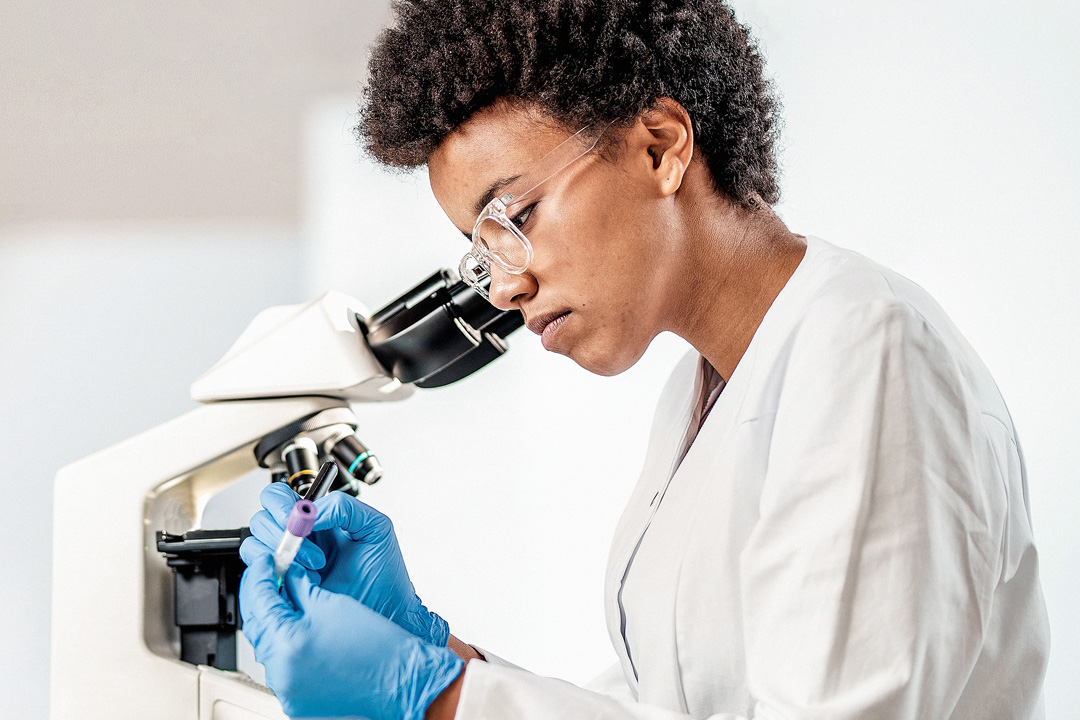
Are You Maximizing the ROI of Your Real-World Data?
Help your organization capitalize on these vital resources using lessons and tools compiled by the Optum life sciences real-world data team.
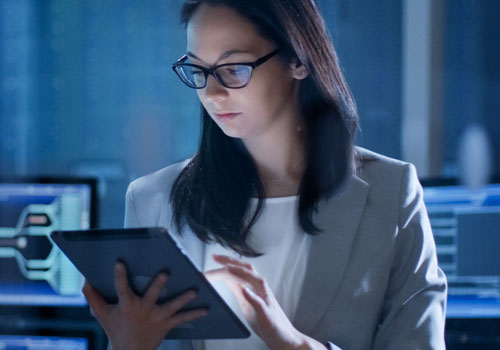