Louis Brooks,
Senior Vice President of Real-World Data and Analytics, Optum Life Sciences
Eric Fontana,
Vice President of Client Solutions, Optum Life Sciences
It’s an exciting time to be working with real-world data (RWD). For life sciences companies, the data’s potential to accelerate learning and drive impact continues to expand. Through our work with a diverse group of more than 100 life sciences organizations across the past several years, we’ve picked up on some surprisingly common challenges that frequently befall teams working with RWD, which in this context includes claims data, electronic health records (EHR) and assets formally linking those 2 sources together.
Our first clue that a client may have fallen into one of these common real-world data pitfalls is an expression of surprise at the nature of the raw data or anomalies that might be found within a real-world data set. While no RWD asset is perfect, we’ve worked with enough life sciences teams to know that these comments most often stem not from poor quality data, but rather from misunderstandings and misapplications of the underlying data assets. And those misunderstandings can lead to confusion and inefficiencies that hinder the 2 overarching imperatives for life sciences data and analytics executives today:
Maximizing return on data investments
- As sources of RWD increase, more RWD vendors offer their latest tools and technologies, and the nature of health care delivery itself continuously evolves, the data available to life sciences companies is becoming more fractured.
- Understandably, data executives want to assess the value of their existing data licenses relative to these new sources. Unfortunately, very few organizations we’ve encountered have well-established methodologies for evaluating the return on investment (ROI) of these data assets in ways that will meaningfully help them understand value beyond simple metrics. There are several key factors organizations must think about to improve ROI.
Confidently explaining data sources and applications to FDA
- Our understanding of FDA guidelines on the way they assess real-world evidence (RWE) in regulatory decision-making continues to mature. It’s clear that manufacturers must be able to substantiate the quality and provenance of the evidence they submit for review. With this extra level of scrutiny, data leaders are asking their data vendors more detailed questions up front in the hopes of avoiding this pitfall and confidently submitting more RWE-informed studies to the FDA.
Together, these 2 imperatives shape a lot of the conversations we’re having with life sciences leaders. To the extent that the common RWD challenges we’re seeing can also hinder progress against these goals, it’s essential that real-world data buyers and users know how to identify and overcome these data pitfalls.
Over the course of this article series, we’ll walk through 7 of the most common pitfalls. By illuminating these challenges, we hope to help you improve the efficiency and effectiveness of your RWD initiatives, ideally improving the return on your data investments as well.
Pitfall 1: Not using data that are fit for purpose
As we look at effective ways to approach data, the first common pitfall we’ve identified is not using data sets that are fit for purpose. Similar to the old saying, "wrong tool for the job," you might be able to use some of the data you have, but your results won’t be as impactful or efficient as if you’d employed the right tool from the beginning.
The first sign of a potential fit-for-purpose problem: When someone claims that their real-world data may have surprised them, which can happen for many reasons:
- The population in the data set may be wrong or incomplete.
- The outcome of interest may not be captured in the data at hand.
- The question could have been answered more comprehensively using clinical or integrated data, rather than claims data alone.
- The cost information is absent when using EHR or registry data.
Too often, data users perceive this to be a data quality issue when, in fact, they’re just using the wrong data to answer the question at hand. Even when you’re starting with the right RWD research question, it’s critical to ask the corresponding data question up front: Does this asset provide us with the data we need to answer the question(s) we’re asking, or to confirm the hypothesis we’re testing?
Recommendations that ensure RWD fitness for purpose
- Understand the composition of the data asset and whether the elements within it enhance or limit the ability to address your central question. Ask yourself, what key data elements are necessary to answer the underlying business questions? Then, build a rubric that includes the specific clinical measures, biomarkers, lab results or financial metrics that are required to answer those questions. As you compare your available data sources, you may need to make trade-offs based on what data are captured or the available patient counts in each attribute.
- Distinguish between the must-haves and the nice-to-haves. In parallel, ensure your key stakeholders are aligned with any gaps in the data before you proceed.
- Evaluate in advance. Distinguish between the elements that are essential to addressing research questions versus the overall depth and breadth of the data, both from a patient and care pathway perspective.
Pitfall 2: Not starting with a specific question
The second pitfall happens more often than you think, and it typically starts with analysts taking an unmapped “walking tour” of the data asset to see what it contains and assess its utility for research purposes.
We've all been there before — exploratory data analysis is one of the first concepts a prospective data scientist learns. It taps into our inner research nerd and that sense of childlike wonder. It’s a new data toy we want to open and play with, often by running counts or queries, just to see what’s inside. But at a certain point, business imperatives must take priority, and the researcher must ask, "What question am I actually trying to answer here?"
If there is no obvious question guiding the scans, counts and queries, that should trigger a moment of self-awareness — it’s likely time to pause the RWD research and take a different approach.
That pause is critical because, in most instances, it's nearly impossible to understand the value of a particular data set without a guiding question to orient the overall exploration. An unguided walking tour of any RWD analyses can lead to a mischaracterization of the data set, which down the road may discourage the unsuspecting researcher or stakeholder from using it.
In many situations, researchers mistakenly conclude that a real-world data set is not usable. But in fact, it may be very valuable once the research questions are appropriately defined.
Researchers and leaders can overcome this almost instinctual pull toward wandering around the data to assess its value and instead navigate through it based on predetermined destinations.
Recommendations for navigating RWD research
It's easy to get lost in the weeds. And not every data set meets every need. But you also can’t fully assess value without a clear sense of what you need to learn. Before proceeding with data licensing or exploration:
- Know what question(s) you want to answer.
- Know what data elements or outcomes should be measured.
- Know how you plan to use what you learn.
Questions to ask your organization and data partners
Avoiding these pitfalls will increase the efficiency and effectiveness of your RWD initiatives, which will improve ROI and bolster confidence in any FDA submissions.
To minimize the risk of falling into these common traps, start by asking yourself and your organization about existing capabilities. Unearth the potential red flags before they become barriers to effectively using the RWD tools you have today or investing in the RWD tools you’ll need tomorrow. In our experience, almost all life sciences organizations could benefit from reflecting on these questions at some level:
- Are we defining our RWD questions ahead of time and developing knowledgebases of our data sets internally, to ensure licensing is appropriate and nonduplicative?
- Are we developing methods to investigate the analysis of RWD data specific to the needs of a given project?
Ask your potential data partners:
- Do you teach licensees to use the data well in advance of a critical business need?
- Do you provide easy-to-read resources to expedite learning, such as FAQs, data explainers and more?
We’ll continue to explore more common pitfalls throughout this article series, including ways to reconcile your RWD expectations and understand the full patient journey. In sharing these perspectives, we hope to create a dialog around the lessons of others, ultimately helping you maximize the return on investment of your real-world data.
Related content
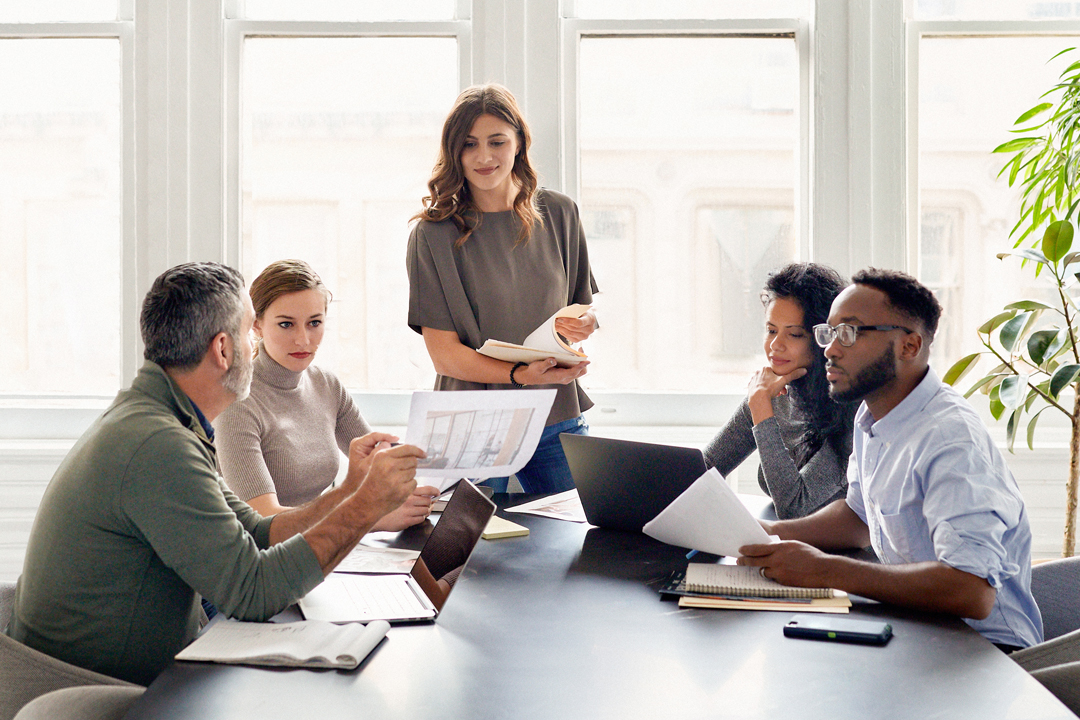
Reconcile your RWD expectations to maximize your investment
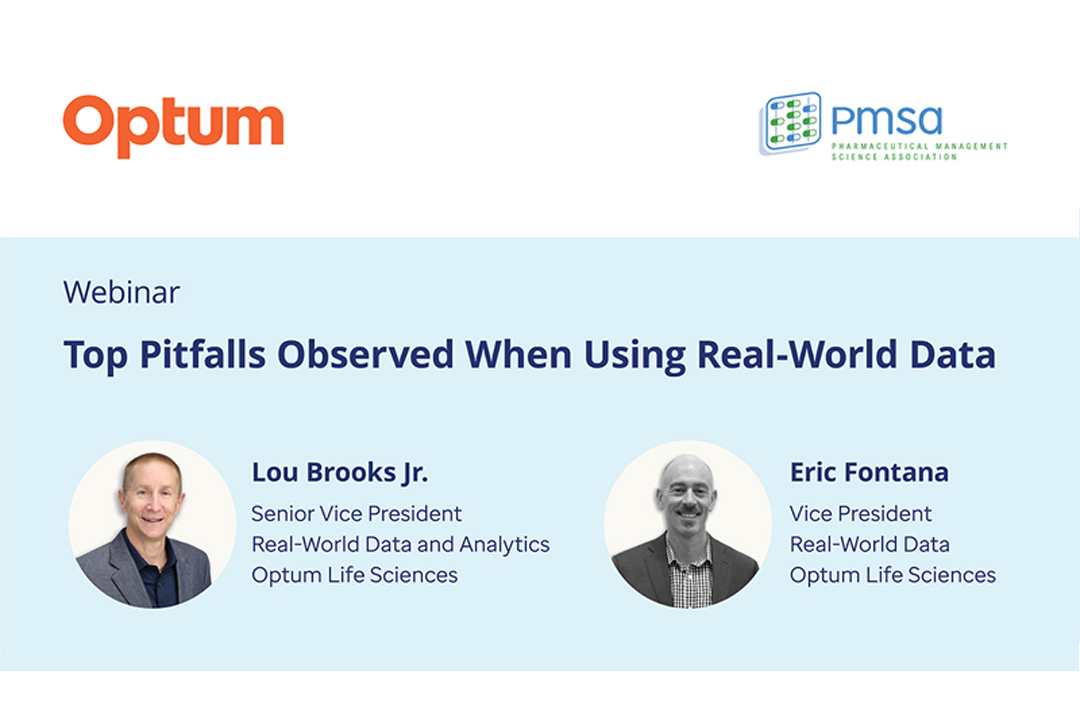
PMSA Webinar: Avoiding common RWD pitfalls
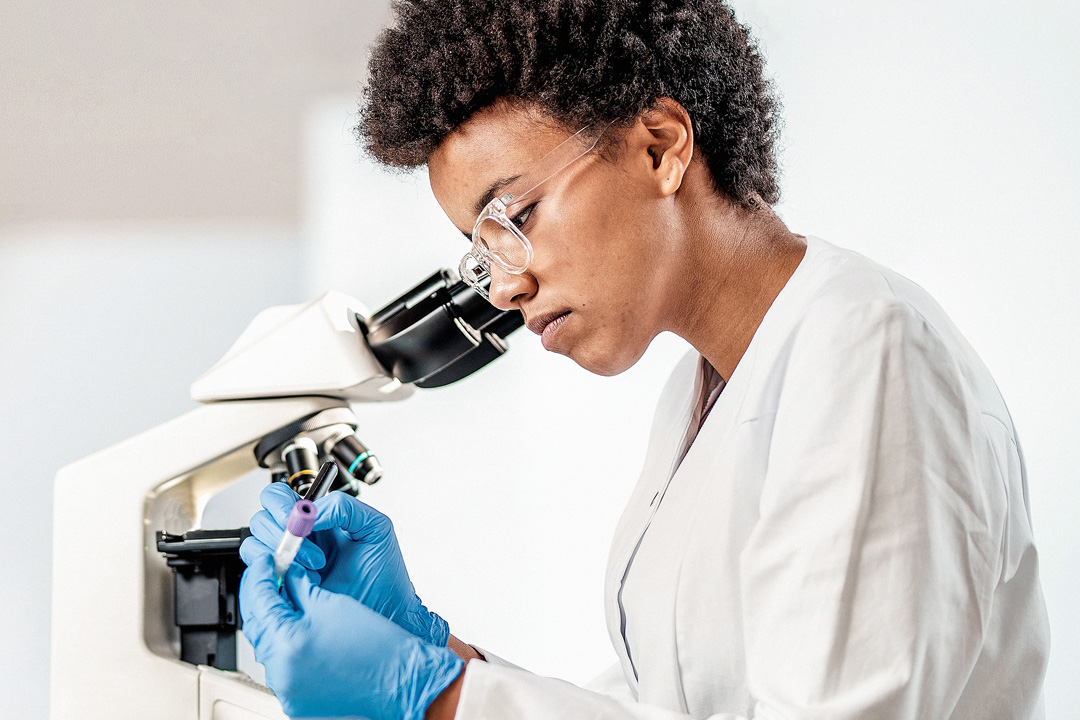